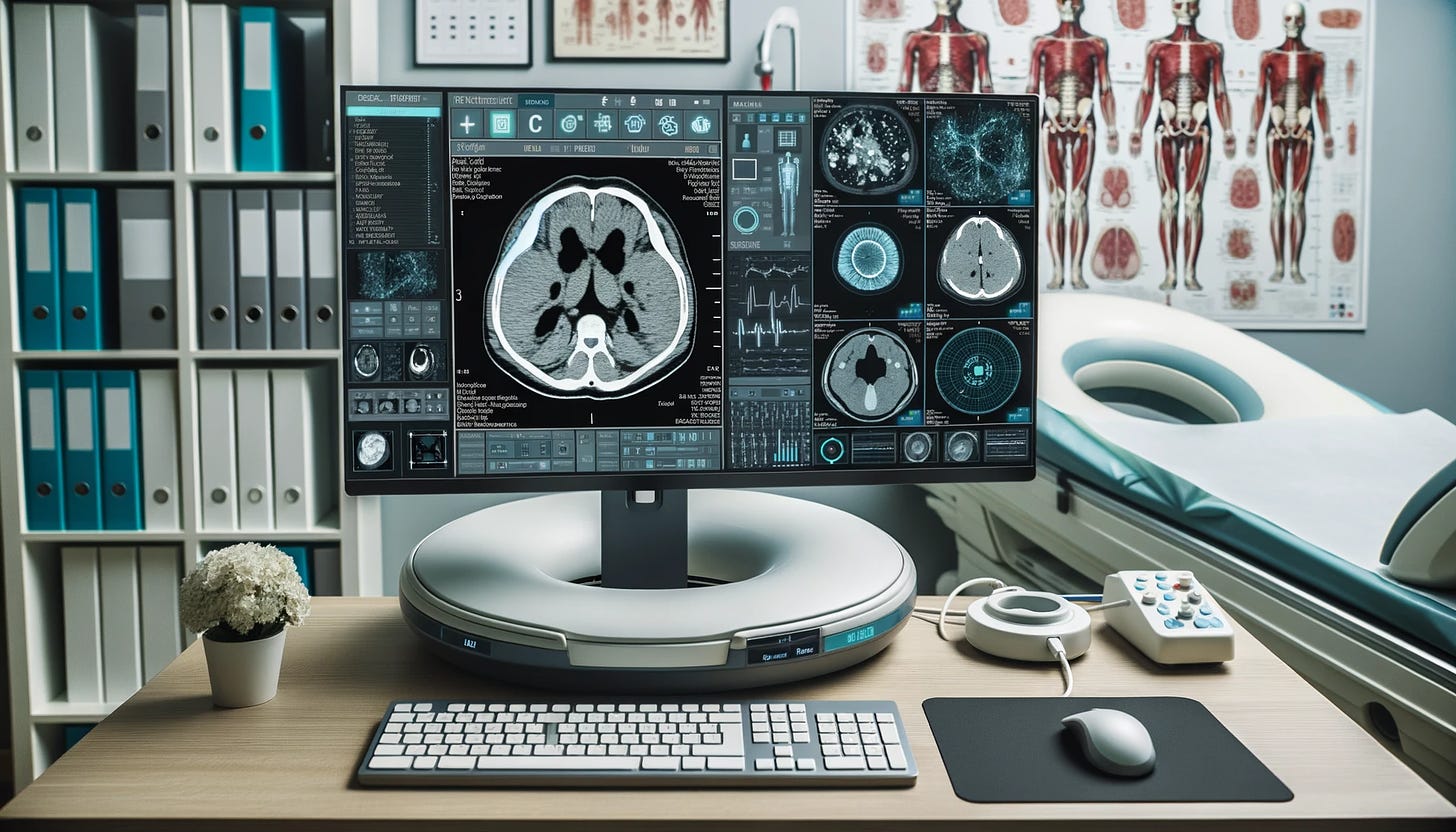
Following my recent post on whether or not AI will replace pathologists and radiologists, I thought it would be a nice segue to talk about how that AI-generated advice is perceived by clinicians, and to combine it with the new “5-Minute Paper” format. Setting aside inaccurate algorithm performance, there is a risk that doctors will simply “turn off their brains” and trust the automated advice, assuming it to be superior to human experience or intuition. So today I’m going to walk-through a recent study that tested this possibility.
Keep reading with a 7-day free trial
Subscribe to All Science Great & Small to keep reading this post and get 7 days of free access to the full post archives.